Results for the Salem/CSPI Prediction Tournament
The problem with prize markets, and what makes a good forecaster
Thanks to everyone who participated in the Salem Center/CSPI forecasting tournament (see here for rules and background). Overall, 1,491 accounts were created. Of those, 472 bet at least S$50 on at least 5 different markets, and 272 met the same threshold for at least 10 markets. A total of 548 individuals filled out the attached survey, with questions related to respondents’ backgrounds, demographic information, and political and social views. Of those, 527 were able to be matched to people who participated in the tournament.
The winner of the $25,000 prize, and the Salem Center fellowship for 2023-2024, is Jonathan Zubkoff, better known to other participants as zubbybadger. We also had a $5,000 award for the person who most improved their portfolio between February 15 and July 31, and the winner was Malte Schrödl, a college student studying in Aachen, Germany. Winners of the Gold, Silver, and Bronze prizes have all been notified, as have the top 20 finishers, who will be invited to a forecasting tournament taking place at the Salem Center in the fall.
The second place finisher in the tournament was at the end going by the handle “guyin2ndplace.” He’s written a very interesting account of his experience. See Part I and Part II. For other commentary on the tournament, see Astral Codex Ten. One can browse the history of all of the markets here, and get historical data related to activity on the site here.
This report is meant to provide a summary of what we have learned. Part I deals with the issue of calibration. How good were our betting markets at actually predicting the future? The rest of the report looks at the participants themselves. Part II gives summary statistics on their backgrounds and political views. Finally, Part III discusses what variables correlated with being a good or bad forecaster.
Data used for the calibration analysis is being made available at the bottom of this report. Most of the data related to the characteristics of bettors is being kept under wraps due to privacy concerns, but we are open to collaborating with those who would like to explore the results. All of the analysis below should be taken as preliminary. We invite others to look for further insights that can be found in the data.
I. How Good Was the Market?
I start by taking the beginning of day probability for each market and each day, and then finding an average for each market across the entire time period. From that, I calculated a Brier score for each individual market. The average across all markets was 0.133. The biggest misses were on the following questions, listed with their average probability and eventual outcome.
Republicans favored in Senate on Election Day?: 34.6% chance, settled YES
Paul Vallas mayor of Chicago?: 63.9%, settled NO
Biden cancels student debt in 2022?: 38.1%, settled YES
Will PredictIt survive?: 38.6%, settled YES
Michigan abortion rights landslide?: 57.1%, settled NO
I don’t see much of a pattern here. One might’ve thought that the market would be biased in the direction of the political leanings of participants, which are discussed below. But they are more conservative than liberal, and their biggest miss was not believing Republicans would be favored in the Senate on election day, when in fact they were. I also assume that participants wanted PredictIt to survive, given that they were presumably supporters of prediction markets. Overestimating Paul Vallas could’ve been a mistake based on their political and class orientation, but the upset in the Chicago mayoral race seems to have been something a lot of people missed.
For the next analysis, we take the first probability of each market every day across the whole tournament. This gives us 12,521 observations. Since there were 91 markets, that means each market existed for an average of just under 138 days.
I divide the observations into 99 bins, from 1% to 99%, and then calculate the average outcome. For example, the market on whether California would ban flavored tobacco was at 58% at the beginning of the day on August 23, and that market ended up settling as YES. Thus, the 58% likelihood was one observation and the dependent variable connected to it was 1. That observation was grouped in with all other observations that rounded to a 58% likelihood, and this was done for each observation in the dataset.
The results are in Figure 1 below. Dotted lines show 95% confidence intervals as calculated using the Adjusted Wald Technique developed for data with binomial outcomes.
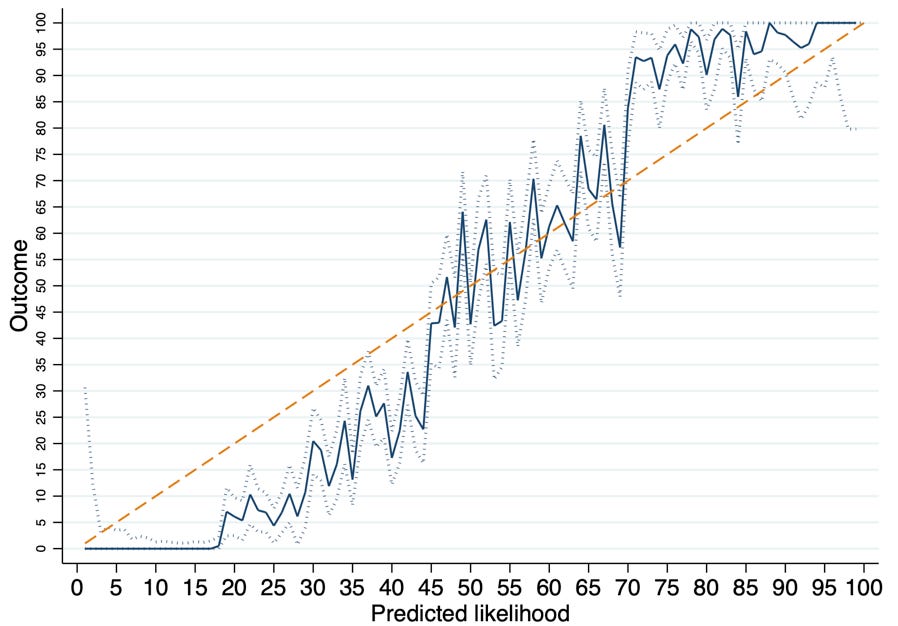
Before the start of the tournament, it was known that by having prizes given at the end, individuals would have an incentive to bet on longshot probability events. Since there’s no advantage to being at, say, the 50th percentile over the 10th percentile, participants would have been smart to take large risks.
Figure 1 shows that this is exactly what they did. Of the 2,504 datapoints for which the market gave the outcome a 17% chance or less of happening, the market resolved YES exactly 0 times. For outcomes that the market gave a 20% chance of happening, the outcome was YES only 6% of the time.
The orange line in Figure 1 represents perfect calibration. Ideally, the blue line would hover around it. But it doesn’t at any point cross the perfect calibration (orange) line until the predicted probability of events is 47%. For high probability events, at every point on the x-axis beginning at 70% each bin had outcomes that were more likely to settle as YES than what the market predicted.
Basically, the market lets you divide events into four categories. Things that are given a 20% or less chance of happening have close to a 0% chance of happening in the real world. Between 20-45% the market becomes more useful but systematically underestimates the likelihood of events. Only between around 45%-70% does the market behave as one would wish. Anything that is given a higher probability than that is close to a sure thing. The problem with betting on longshots seems to be worse at the left side of the graph. In other words, people like the idea of a longshot YES bet over a longshot NO bet. There is probably a reason for this out there in some psychological literature. Or is this perhaps due to non-randomness in how we framed the questions, that is, which outcomes we chose to call YES and which we called NO?
This is pretty bad relative to real prediction markets. Here’s one study of PredictIt, based on prices one month before resolution.
One sees the same problem, with the calibration line being below the perfect calibration line at low prices and above it at higher prices. Nonetheless, this is clearly nowhere near as bad as our own chart. Markets that are based on prizes, like ours was, are clearly not useless. Since the errors are somewhat predictable, they provide good information. One can assume that things are correctly priced near the center of the probability distribution, overpriced at the low end, and underpriced at the high end. We are therefore left with data supporting the well-established but nonetheless important conclusion that prize markets are better than nothing, with markets based on money, or some adequate substitute that doesn’t over incentivize longshot bets, being much superior for predicting high and low probability events.
II. The Bettors
We had 548 individuals take the survey. Of those, 95.1% were male, compared to 4.3% female, and less than 1% other. It was a young crowd, with 48.8% aged 28-42, and another 35.7% aged 27 or younger. 88.9% were straight, with 5.5% bisexual.
Just under 74% were from the United States, and close to 85% identified as white. The sample was extremely secular compared to the general population, with 53% identifying as atheist or agnostic. 77.8% had graduated from college, and 39.7% had some kind of post-graduate degree.
The most common fields were tech (24.7%), academia (grad student or research fellow) (11.4%), government (7.7%), academia (professor) (6%), entrepreneur (non-tech) (6%), academia (other) (5.8%), nonprofit (5.6%), banking (5.2%), tech (business owner) (5.2%), politics (2.8%), and think tank (2.8%).
Individuals were asked to describe themselves politically on a five-point scale, from very liberal (1), to moderate (3), to very conservative (5). The survey takers rated themselves on average 3.26 on economic issues, 2.79 on social issues, and 3.07 overall. In other words, the sample can be described as broadly libertarian leaning.
More precisely, the field can be described as pro-democratic capitalism, of a techno-optimist flavor.
We know this because we asked respondents to rate 78 different ideas, people, and concepts, from Donald Trump to bringing back dinosaurs to embryo selection to banning pornography.
The four statements and ideas they rated most favorably were “I believe people are better off than at any other point in history” (4.07 on a 5-point scale); “I believe capitalism is a better economic system than all available alternatives” (4.07); “I believe anthropogenic climate change is real” (4.01); and “Democracy” (3.72). The least popular were “There should be no law against a boss pressuring an employee for sex” (1.82); “It is more likely than not that the Democrats stole the 2020 election” (1.85); “I believe humans have made contact with aliens” (1.85) (note this was before that topic started to be in the news); and “I believe people in developed countries should have fewer children” (1.89).
As found in previous studies of this kind, participants were more likely than chance to be first borns. 55.1% of respondents reported having no older siblings, compared to 34% who reported no younger siblings. With a sample size of 492 individuals answering both questions, this result passes all conventional thresholds of significance.
III. What Makes a Good Forecaster?
Figure 3 shows the twenty statements with the strongest correlation, positive or negative, with being a good forecaster, defined as the balance in S$ an individual ended up with. For this analysis, I only included bettors who placed at least S$50 on 5 different markets and answered all item questions, of which there were 192 individuals.
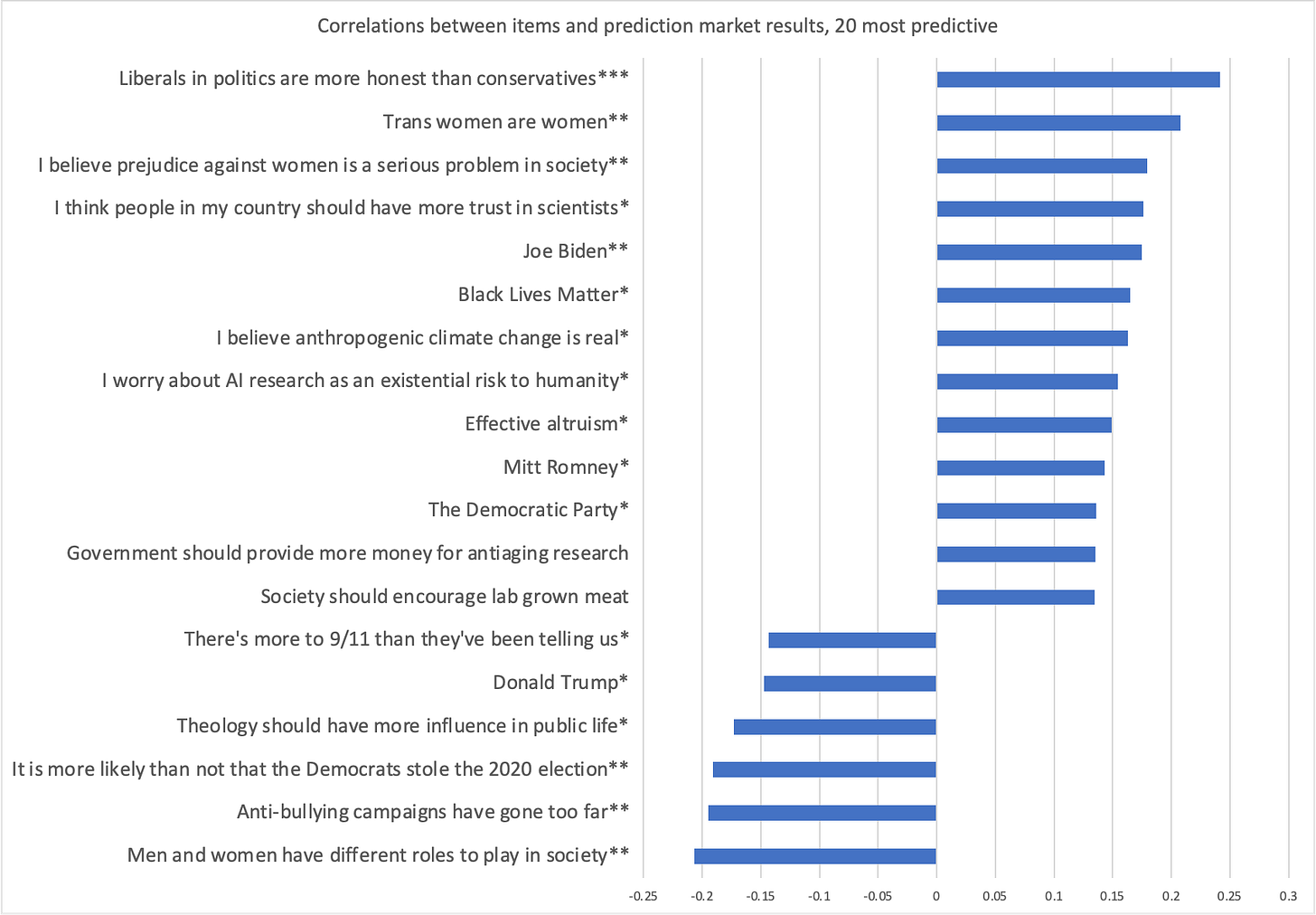
If there’s one takeaway from the chart above, it’s that the best forecasters liked left-wing ideas, people, and movements. The best predictor was agreeing with the statement that liberals are more honest than conservatives. Since I’ve written articles to this effect, this warmed my heart. But that result was just part of a general pattern of liberals being better forecasters than conservatives. A univariate regression likewise shows that those who identified as more conservative on the five-point scale ended up with lower balances, with the result just missing the conventional threshold of significance (p = .056).
There are two plausible theories here, other than the possibility that liberals are just superior at predicting the future. First, since this was a highly selected group, it may have been that liberals who participated in the Salem/CSPI tournament were of a higher quality, because they were familiar with and willing to participate in an event that was coded as being ideologically opposite of them. Recall that the crowd leaned more conservative overall, albeit slightly. For reference, participants rated BLM (2.46) higher than Biden (2.34) and Trump (2.1), but lower than libertarianism (3.21) or DeSantis (2.86). The Democratic Party (2.06) and the Republican Party (2.08) were rated about equally negatively.
The second possibility is that the liberal advantage is explained by the results of the midterms. Of our 91 markets, 18 had to do with the American elections held in November 2022. Imagine if each side tended to bet on how they wanted things to turn out. Then liberals would have ended up doing much better, because Democrats outperformed the conventional wisdom. PredictIt gave Republicans a 74% chance of winning the Senate on the day before the election, and the FiveThirtyEight model ended up at 59%.
This led me to wonder, what happens if you simply take out the 18 midterm markets, and see how people did in the other 73 markets? Figure 4 shows the results for the top 20 items most strongly related to forecasting outcomes not having to do with the midterms, otherwise using the same methodology as described for Figure 3.
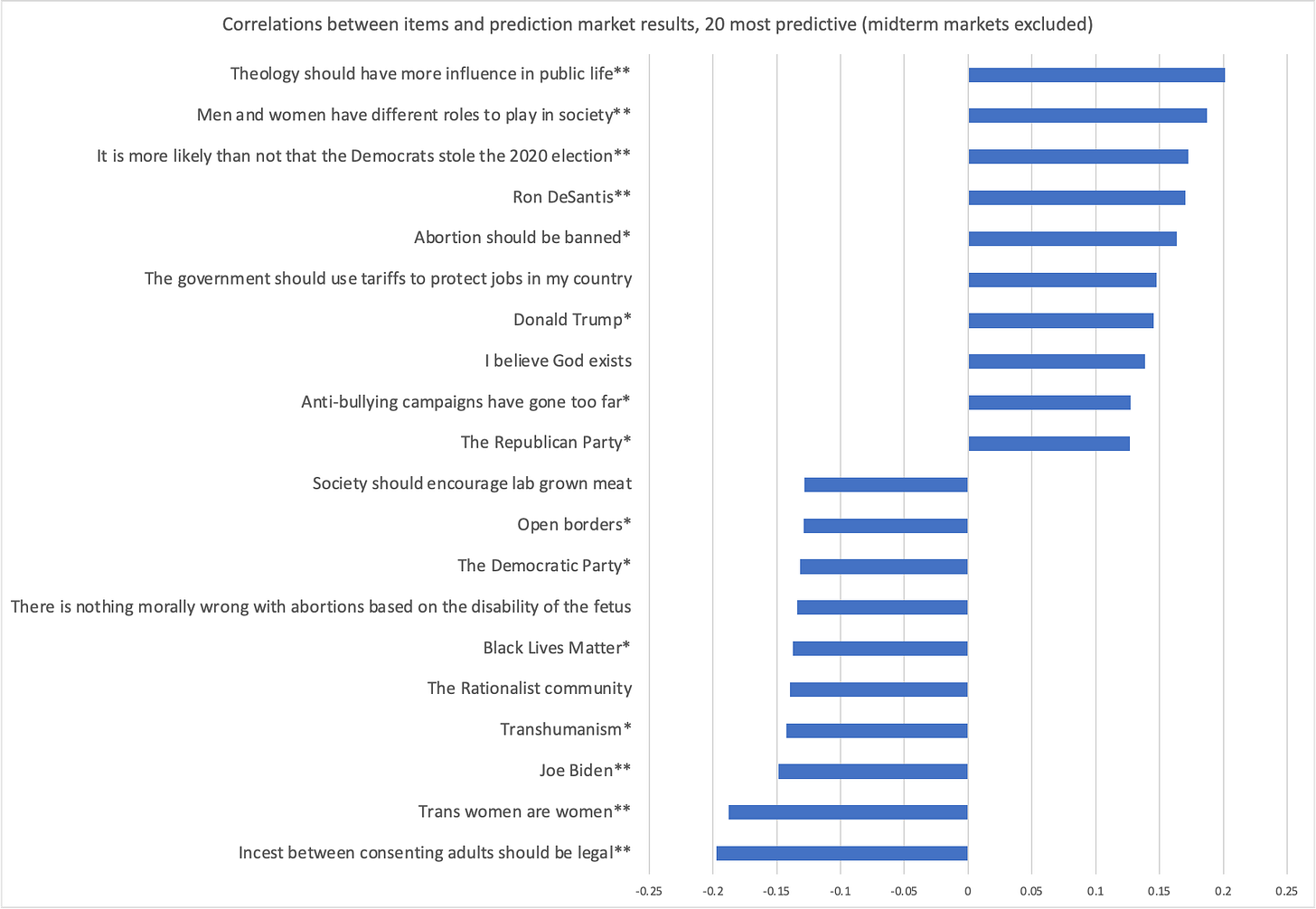
Suddenly, conservatives are the better forecasters. If you want someone’s advice, you should look for an individual who thinks theology should dominate public life, incest definitely shouldn’t be legal, and Trump was robbed in 2020. Unless, that is, you want to predict the 2022 midterm results. The results in Figure 3 and Figure 4 are not driven by outliers. Removing the biggest winners of the tournament does not change the pattern of liberal respondents doing better overall and conservatives performing better in non-midterm elections.
For both Figure 3 and Figure 4, there is an obvious potential multiple comparisons issue. When a Benjamini-Hochberg FDR correction is applied to the p-values, 7 items remain significant in Figure 3 and 5 in Figure 4.
Is the conclusion that nobody is better than anyone else? Mostly, yes. The relationships found are quite weak, with very few items having a correlation of at least r =+/- 0.2 with forecasting results. Yet I think we do have to give the advantage to liberals. It would be unfair to discount their accomplishment just because much of it was driven by the 2022 midterms. If conservatives were too optimistic about their own side winning, then that is their fault. Maybe Democrats would’ve been too optimistic about their chances in a different year, but we can’t just assume that they would have been. So credit to the left leaning participants, but keep the two caveats in mind that they didn’t do as well in non-midterm markets, and the ones who participated in this tournament are a highly selected group. While the midterm story can explain the overall results, it doesn’t provide an obvious hypothesis as to why conservatives would be better in non-midterm markets.
Data
I’d like to encourage anyone who is interested to explore the data for themselves. Please reach out if you do at contact@cspicenter.org, as we would be interested in hearing about and publicizing your results. All of the analysis in this report was done in Stata or Excel. The spreadsheets to reproduce the data in Part I can be found below, along with the codebook, explaining what’s in each file. As mentioned above, we’re withholding most of the data relied upon in Part II and Part III, as it can be used to identify individuals and gain access to personal information. We are, however, making available the ratings of various items and political self-identification in the file Salemratings below. If you would like to test hypotheses about any other individualized data, contact us and we can possibly discuss some kind of collaboration or non-disclosure agreement.
All files listed below can be found here, in addition to a .json file with all the bets.
Great writeup, very interesting results! Congrats on completing it, and congrats to the winners.
I work at Manifold and helped run the tournament. Was surprised that political leaning was so important to the results!
The final calibration was definitely underwhelming, but I think it's not all due to the effect of people trying to take risky bets to win the top prizes. Another factor was a limited supply of currency with which to bet.
One condition of the tournament was that everyone began with a fixed amount of currency: S$ 1000. Each market has an AMM (automated market maker) which was also started with about S$ 2000 liquidity. As a player, you might not want to spend all your balance moving the probability from 85% to 95% if you could make more profit in another market. On the main Manifold site, you can buy more currency, and also there are automatic loans that return you your currency to bet again, which both help.
See also "Opportunity costs are critical" in this writeup: https://blog.polybdenum.com/2023/08/01/how-i-came-second-out-of-999-in-the-salem-center-prediction-market-tournament-without-knowing-anything-about-prediction-markets-and-what-i-learned-along-the-way-part-1.html
The effect of limited currency meant users did not bet probabilities as far as they would have, which matches the worse calibration at the extremes.
What's your explanation for why, aside from the midterms, conservatives did better? Was there some event where Republicans did surprisingly well which you should also exclude?